Bachelor Theses
Bachelor theses in Statistics consist of a description of new statistical methods and their application on specific data.
-
Registration
We would like to ensure that all students of the Economics and Management bachelor receive a topic for their theses within standard period of study. Therefore, allocation is made by the Office of the Dean of Studies via a centralized application procedure. Afterwards, the Office of the Dean of Studies will inform you about the institute which you were assigned to.
-
Assignment of Topics
We have prepared a selection of topics for students who were assigned to our institute. You can find a list with all current topics on our website.
Topics for summer term 2024 as well as winter term 2024/25 will be assigned as desired within the available topics. Therefore, please contact the office with your desired topic by email.
AvailabilityAs soon as you have told us about your chosen topic, we will reserve it for you.
Topics
We have arranged a selection of topics from different areas.
In the following, you may find a list of currently available topics which may help for your choice.
-
Topics
Heteroskedastizität
Im klassischen linearen Regressionsmodell wird eine konstante Varianz der Fehlerterme angenommen. Bei sich verändernder Varianz ist der Kleinste-Quadrate-Schätzer nicht mehr effizient. Getestet wird dies z.B. mit dem White-Test oder dem Breusch-Pagan Test (Original oder Koenkers Version). Als Lösung werden Heteroskedastizität-robuste Standardfehler oder die Gewichtete-Kleinste-Quadrate-Methode genutzt. Falls gleichzeitig Autokorrelation vorliegt, finden sogenannte HAC-Schätzer Anwendung (heteroscedasticity and autocorrelation consistent).
Einstiegsliteratur:- J.M. Wooldridge. Introductory econometrics: A modern approach. Nelson Education, 2013 (Kap. 8 + 12)
- W.H. Greene. Econometric analysis. Pearson Education, 2012 (Kap. 9)
Autokorrelation
Die Regression mit Zeitreihendaten könnte dazu führen, dass klassische Annahmen an den OLSSchätzer verletzt sind, sodass dieser nicht mehr effizient ist. Autokorrelation ist ein Beispiel dafür. Wenn Autokorrelation vorliegt, sind die Fehler einer linearen Regression zeitlich abhängig. In dieser Arbeit soll das AR(1) Fehlermodell vorgestellt werden. Darüber hinaus sollen Tests auf Autokorrelation vorgestellt werden und aufgezeigt werden, wie man trotz Autokorrelation effizient lineare Regressionsmodelle schätzen kann.
Einstiegsliteratur:- J.M. Wooldridge. Introductory econometrics: A modern approach. Nelson Education, 2013 (Kap. 12)
- W.H. Greene. Econometric analysis. Pearson Education, 2012 (Kap. 12)
Endogenität
Im klassischen linearen Regressionsmodell ist eine Voraussetzung für die Konsistenz des OLS-Schätzers, dass die Kovarianz zwischen der Regressormatrix und dem Fehlerterm Null ist. Falls diese Annahme verletzt ist, liegt sogenannte Endogenität vor. Eine Folge davon ist, dass der OLS-Schätzer einen Bias besitzt. Eine Möglichkeit, um mit Endogenität umzugehen,ist die Verwendung von sogennanten Instrumentalvariablen. Diese werden mithilfe des Two Stage Least Squares (2SLS) Verfahrens geschätzt, um eine konsistente Schätzung der Koeffizienten zu erlangen. Das Thema kann um eine weitere Lösungsmöglichkeit für das Endogenitätsproblem erweitert werden: Das Prinzip der generalisierten Momentenmethode (Generalized Method of Moments, GMM) liegt in der Festlegung von Bedingungen für die Momente der unterstellten Verteilung der Störterme des Modells. Die zu schätzenden Parameter werden so gewählt, dass sie möglichst gut im Einklang mit den Bedingungen stehen.
Einstiegsliteratur:- J.H. Stock und M.W. Watson. Introduction to Econometrics. Pearson Education, 2011 (Kap. 12)
- J.M. Wooldridge. Introductory econometrics: A modern approach. Nelson Education, 2013 (Kap. 15)
- W.H. Greene. Econometric analysis. Pearson Education, 2012 (Chap. 13)
- J.M. Wooldridge. “Applications of generalized method of moments estimation”. In: Journal of Economic perspectives 15.4 (2001), S. 87–100
Simultane Gleichungssysteme
Ein einfaches simultanes Gleichungssystem lässt sich dadurch charakterisieren, dass die abhängige Variable in der einen Gleichung als erklärende Variable in der anderen Gleichung vorkommt und umgekehrt. Daher entsteht ein Endogenitätsproblem. Zwei Probleme sollen in dieser Arbeit näher erläutert werden: Zum einen das Problem der Identifikation, d.h. unter welchen Umständen können die Koeffizienten beider Gleichungen geschätzt werden. Zum zweiten sollen Schätzer vorgestellt werden, die unter Endogenität funktionieren und die die Koeffizienten des Systems Gleichung für Gleichung schätzen.
Einstiegsliteratur:- W.H. Greene. Econometric analysis. Pearson Education, 2012 (Kap. 10)
- F. Hayashi. “Econometrics”. In: Princeton University Press (2000) (Kap. 8)
- J.M. Wooldridge. Econometric analysis of cross section and panel data. MIT Press, 2010 (Kap. 8+9)
Treatment Effects
Beim Schätzen von durchschnittlichen Treatment Effects geht es darum den Effekt verschiedenster Maßnahmen wie z.B. einer Weiterbildung zu analysieren. Insbesondere besteht die Frage wie man diese Effekte möglichst genau messen und kausal interpretieren kann, falls keine komplett randomisierten Experimente durchgeführt wurden. Für diesen Fall können Matching Verfahren angwendet werden um möglichst ähnliche Einheiten in der Treatment- und der Kontrollgruppe miteinander zu vergleichen. In dieser Arbeit sollen die zwei prominentesten Matching Verfahren und ihre Eigenschaften vorgestellt worden: Covariate Matching und Propensity Score Matching. Beim Covariate Matching werden verschiedene Einheiten basierend auf ihren beobachtbaren Eigenschaften miteinander gematched. Währenddessen werden beim Propensity Score Matching die Einheiten basierend auf der Wahrscheinlichkeit, dass sie in die Treatment Gruppe gehören, gematched.
Einstiegsliteratur:
- G. Cerulli. Econometric evaluation of socio-economic programs. Springer, 2015 (Chap. 2)
- J.M. Wooldridge. Econometric analysis of cross section and panel data. MIT Press, 2010 (Chap. 21)
Random Forests
Entscheidungsbäume stellen ein leicht zu interpretierendes nichtparametrisches Verfahren dar. Allerdings sind sie in der Praxis oft zu variabel, weswegen meist auf eine Erweiterung, die sogenannten Random Forests zurückgegriffen wird. Diese basieren auf der Idee des Bootstraps. Aus der ursprünglichen Stichprobe wird mit Zurücklegen eine neue Stichprobe gezogen, für die dann ein neuer Entscheidungsbaum bestimmt wird. Dabei wird in jedem Schritt zufällig ausgewählt auf Grundlage welcher Regressoren Entscheidungen getroffen werden können. Dieser Vorgang wird viele Male wiederholt und die Vorhersagen der so entstandenen Bäume werden durch Durchschnittsbildung zu einem Modell zusammengefügt.
Einstiegsliteratur:- G. James u. a. An introduction to statistical learning. Springer, 2013 (Kap. 8)
- L. Breiman. “Random forests”. In: Machine learning 45.1 (2001), S. 5–32
- E. Scornet. “On the asymptotics of random forests”. In: Journal of Multivariate Analysis 146 (2016), S. 72–83
Perzeptron
Das Perzeptron stellt den Grundbaustein moderner neuronaler Netze dar und wird zur Klassifikation verwendet. In seiner grundlegenden Funktionalität kommt das Perzeptron dem multiplen linearen Regressionsmodell gleich. Im Bereich der neuronalen Netze werden die unabhängigen Variablen des Modells als Eingabe in das Perzeptron interpretiert, welche abhängig von den gelernten Gewichten des Perzeptrons zu einer bestimmten Ausgabe führen. Das Lernen der Gewichte erfolgt über einen iterativen Trainingsprozess, dessen Funktionsweise und Limitationen im Rahmen dieser Arbeit vorgestellt werden sollen. In der Arbeit soll weiter auf das Problem der linearen Separierbarkeit der zu klassifizierenden Daten eingegangen und Lösungsmöglichkeiten wie das mehrlagige Perzeptron oder der Maxover-Algorithmus vorgestellt werden.
Einstiegsliteratur:- W. Ertel und N.T. Black. Grundkurs K¨unstliche Intelligenz. Springer, 2016 (Kap. 8.2)
- C.M. Bishop u. a. Neural Networks for Pattern Recognition. Oxford University Press, 1995 (Kap. 3.5)
- F. Rosenblatt. “The Perceptron: A Probabilistic Model for Information Storage and Organization in the Brain”. In: Psychological review (1958), S. 386
Hauptkomponentenanalyse
Die Hauptkomponentenanalyse, oder auch Principal Component Analysis (PCA), dient zur Identifizierung der Varianz-Kovarianz Struktur mittels Linearkombinationen aus den ursprünglichen Variablen. Die generelle Intention dieses Verfahrens dient der Komprimierung der Daten und der Interpretierbarkeit dieser. Bei der Anwendung der Hauptkomponentenanalyse werden häufig Zusammenhänge offengelegt, die vorher nicht offensichtlich sind und daher eine neue Interpretation der Datenstrukturen und Zusammenhänge innerhalb des Datensatzes ermöglicht. Aus diesem Grund wird die Hauptkomponentenanalyse hauptsächlich zur Erkennung von Beeinflussungsmustern und -strukturen in hochdimensionalen Datensätzen besonders im Bereich der Finanzwissenschaft, Data-Mining, Bioinformatik und der Umweltforschung eingesetzt.
Einstiegsliteratur:- R.A. Johnson, D.W. Wichern u. a. Applied multivariate statistical analysis. Prentice Hall, NJ, 2002 (Chap. 8)
- A.J. Izenman. “Multivariate regression”. In: Modern Multivariate Statistical Techniques. Springer, 2013, S. 159–194 (Chap. 7)
- W.J. Krzanowski. Recent advances in descriptive multivariate analysis. Clarendon Press, 1995 (Chap. 5)
- M. Ringn´er. “What is principal component analysis?” In: Nature biotechnology 26.3 (2008), S. 303
Faktorenanalyse
Die Faktoranalyse behandelt multivariate beobachtete Variablen die zumeist von gleichen oder ähnlichen unterliegenden Variablen beeinflusst werden. Ähnlich wie die zu analysierenden Variablen sind auch die zugrundeliegenden Faktoren unterschiedlich für jedes beobachtete Individuum. Allerdings sind die zugrundeliegenden Faktoren unbeobachtbar. Jeder Faktor der verwendet wird erklärt einen Teil der Varianz in den beobachteten Variablen und wird danach geordnet, wie hoch der Anteil der erklärten Varianz von den einzelnen Faktoren ist. Das Ziel der Faktoranalyse ist die Dimensionsreduktion der analysierten Variablen. In der Arbeit sollen vor allem auf die Motivation für Faktormodelle, die Modelldefinition und Annahmen sowie Möglichkeiten der Parameterschätzung eingegangen werden.
Einstiegsliteratur:
- A.C. Rencher und W.F. Christensen. Methods of Multivariate Analysis. John Wiley & Sons, Inc., 2012 (Chap. 13)
- J.F. Hair u. a. Multivariate Data Analysis. Pearson Education Limited, 2014 (Chap. 3)
Clusteranalyse
Die Clusteranalyse wird dazu verwendet um Daten, aus meist multivariaten Beobachtungen, anhand ähnlicher (Verhaltens-)Strukturen in sogenannte Cluster oder Gruppen zu ordnen. Das Ziel ist es optimale Gruppen für die Beobachtungen zu finden, sodass in jeder einzelnen Gruppe nur die Beobachtungen zusammengefasst werden, die eine ähnliche Struktur aufweisen, während die einzelnen Gruppen untereinander keine Ähnlichkeiten aufweisen. Um eine Gruppierung zu ermöglichen, gibt es verschiedene Algorithmen. Die einen betrachten alle Beobachtungspaare die auf Ähnlichkeit basieren. Dabei verwendet die Ähnlichkeitsanalyse eine sogenannte ”Measure of Distance”. Andere Algorithmen verwenden eine vorher festgelegte Clustermitte oder vergleichen die Variabilität der einzelnen Cluster mit- und untereinander. Das Anwendungsgebiet der Clusteranalyse ist vielfältig wie zum Beispiel Medizin, Soziologie, Kriminologie, Anthropologie, Archäologie, Geographie, Marktanalysen, Wirtschaftswissenschaften und Ingenieurwesen.
Einstiegsliteratur:- A.C. Rencher und W.F. Christensen. Methods of Multivariate Analysis. John Wiley & Sons, Inc., 2012 (Chap. 15)
- J.F. Hair u. a. Multivariate Data Analysis. Pearson Education Limited, 2014 (Chap. 8)
k-Nearest-Neighbors
k-Nearest-Neighbors (k-NN) ist eine nicht-parametrische Klassifikationsmethode. Der Grundgedanke ist, einzelne Datenpunkte basierend auf der Klassenzugehörigkeit ihnen ähnlicher Datenpunkte - ihrer Nachbarn - zu klassifizieren. Neben der Definition von Entfernung spielt die Wahl des Parameters k, welcher die Größe der zu berücksichtigenden Nachbarschaft steuert, eine wichtige Rolle. In dieser Arbeit soll zunächst das Prinzip der Nächste-Nachbarn-Klassifikation und ihrer verschiedenen Ausprägungen vorgestellt werden, um darauf aufbauend die Wahl des Parameters k und der daraus folgenden Konsequenzen sowie die Evaluationsmöglichkeiten der resultierenden Klassifikation diskutieren zu können.
Einstiegsliteratur:- W. Ertel und N.T. Black. Grundkurs K¨unstliche Intelligenz. Springer, 2016 (Kap. 8.3)
- C.M. Bishop u. a. Neural Networks for Pattern Recognition. Oxford University Press, 1995 (Kap. 2.5)
Autoregressive Prozesse
Eines der wichtigsten Modelle in der Zeitreihenanalyse ist der autoregressive Prozess (AR), bei dem Beobachtungen anhand von vergangenen Beobachtungen und einem Zufallsschock modelliert werden. Wenn die passende Modellordnung bekannt ist oder geschätzt wurde, also die Anzahl an zu berücksichtigenden vergangenen Beobachtungen, kann mit unterschiedlichen Methoden das Modell angepasst und zur Prognose genutzt werden. Interessant ist besonders die Eigenschaft der Stationarität des Prozesses.
Einstiegsliteratur:- M. Deistler und W. Scherrer. Modelle der Zeitreihenanalyse. Springer, 2018 (Kap. 5)
- K. Neusser. Zeitreihenanalyse in den Wirtschaftswissenschaften. Springer, 2009 (Kap. 2 + 5)
Master Theses
Master Theses in Statistics consists of a description of new statistical methods and their application on specific data. This is similar to bachelor theses. Furthermore, new statistical methods could be described in detail and more critically. Another option is to do an empirical study on a statistical problem.
-
Registration
For master theses allocation is made on student's requests. If you would like to write your master thesis at our institute, you may contact Prof. Dr. Sibbertsen by email.
-
Topic Assignment
Topics for master theses are very diverse. They range from methodical work (method presentation, method comparison, method development) to own empirical work (data collection and analysis) with references to all other economic elective courses.
Topic assignment takes place in coordination with you. We will gladly consider your suggested topics.
Notes for Theses
Below you may find informationen about requirements for bachelor theses as well as a template for LaTeX. Your bachelor thesis should be 15 pages long.
For LaTeX beginners we recommend to use the university’s Overleaf cloud service (https://www.luis.uni-hannover.de/de/services/speichersysteme/dateiservice/cloud-dienste/overleaf/ ). To start with, our template can easily be uploaded as a new project. Otherwise, proper installation of MiKTeX (https://miktex.org/) and a LaTeX editor (https://www.texstudio.org/ ) is required.
Entries for the bibliography file can be copied from Google Scholar (Cite -> BibTeX) or generated with e.g. https://www.doi2bib.org/. In case of many references, a software for reference management (e.g. Citavi, https://www.luis.uni-hannover.de/de/services/betrieb-und-infrastruktur/software-lizenzen/software-katalog/produkte/citavi/) might be useful.
Contact for general questions about your thesis

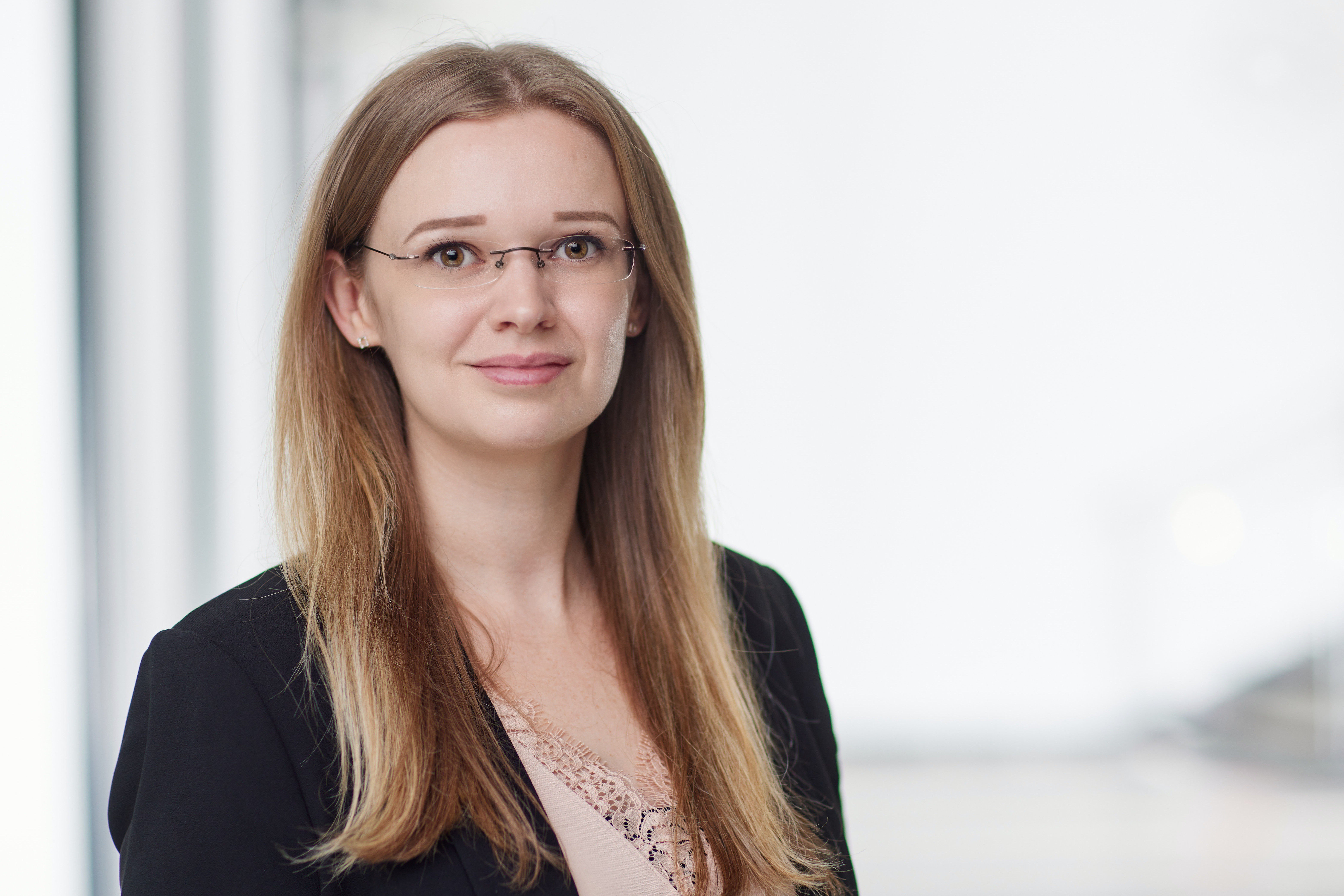
30167 Hannover

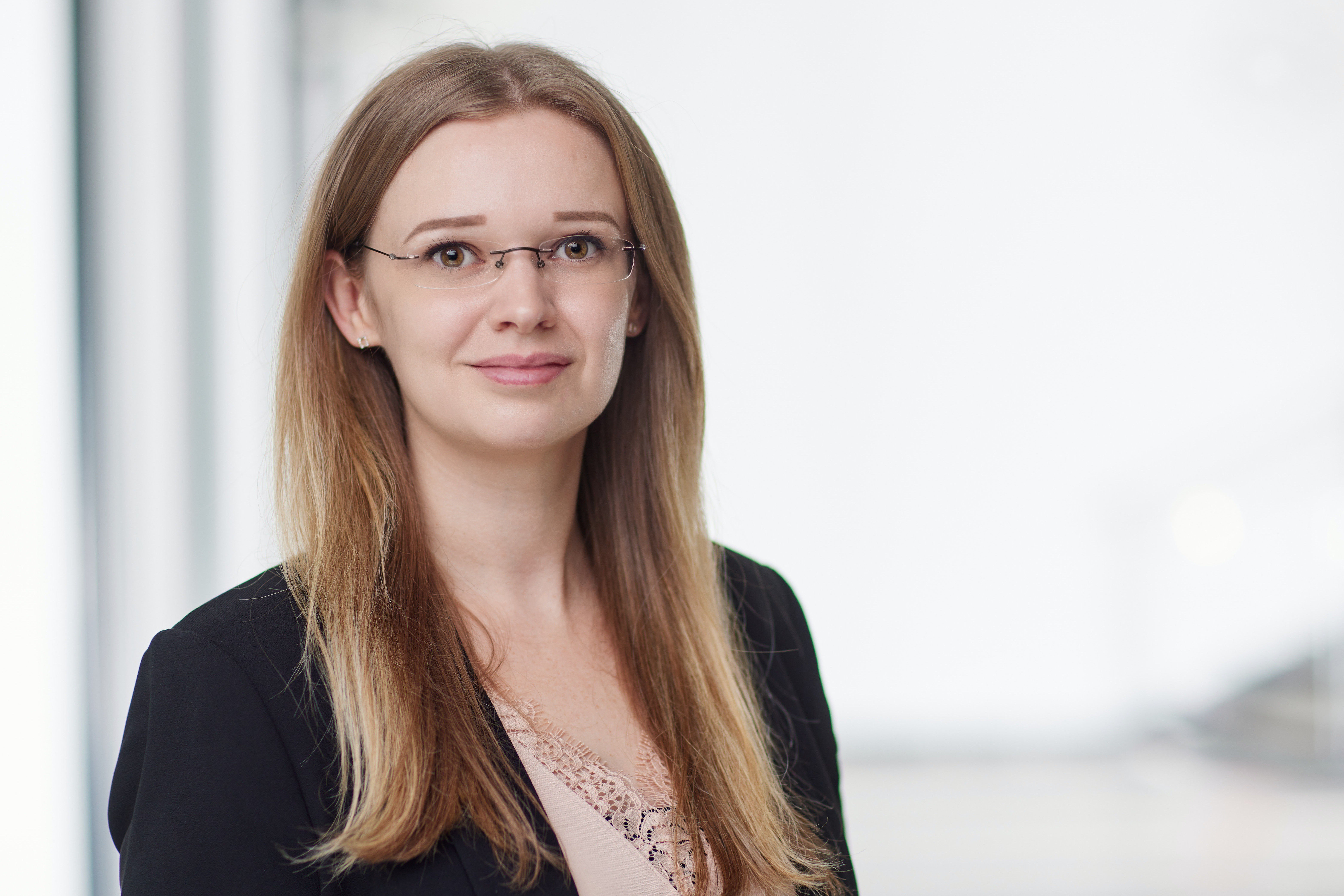